CHALLENGES
- Sources around 700-800M€ of plastic yearly, mainly polyethylene (as P.E.T.)
- Plastic price is volatile, strongly correlated with oil price, leading to high impact on P&L and product margin
- Current process to determine purchase policy is long, manual, lacks of efficiency
- Objectives : take better buying & hedging decisions .
- Busines Case Estimated gain at 10M€/yr
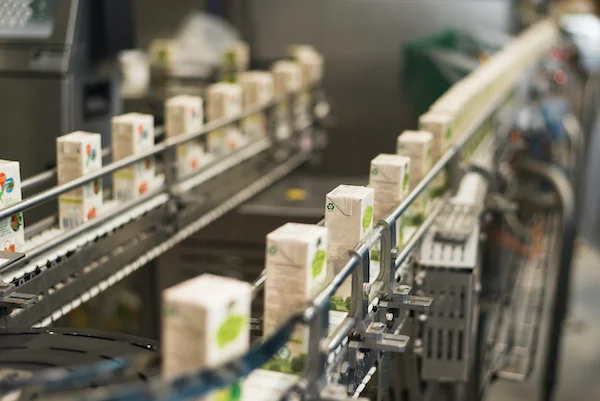
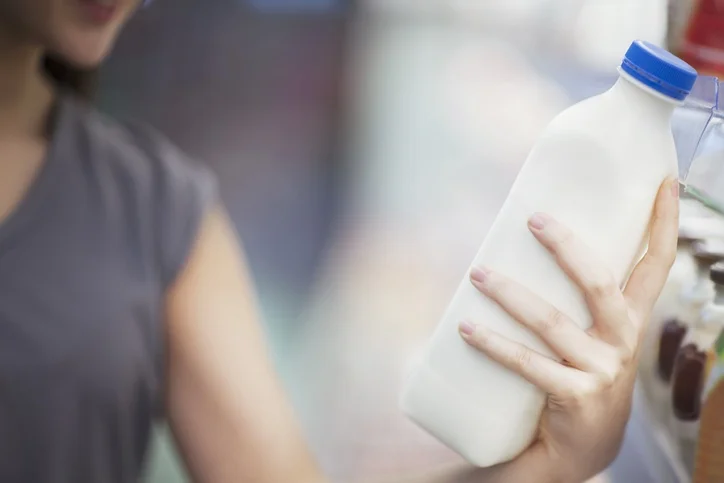
SOLUTION
- Automate market data collection on referent sites (+20)
- Standardize and cleanse data for compliance and consistency
- Build a Datawarehouse for all these values
- Select and parameterize the datascience algorithms best suited to determining prices and forecasting trends
- Have the approach and calculations validated to gain the confidence of the business lines
- Systematically present the results in forms adapted to the culture and needs of the business lines.
BENEFITS
- On-time and trustable insight on the raw material market
- Capability to quickly extend the model to new sources for PET, but to other raw materials as well
- Strong Machine Learning capabilities through XGBoost algorithm
- Historical and comparable database for pricing knowledge and tracking
- UX oriented presentations for a common and trustable signe view of prices truth
- Estimated gain of 7M€ in the first year
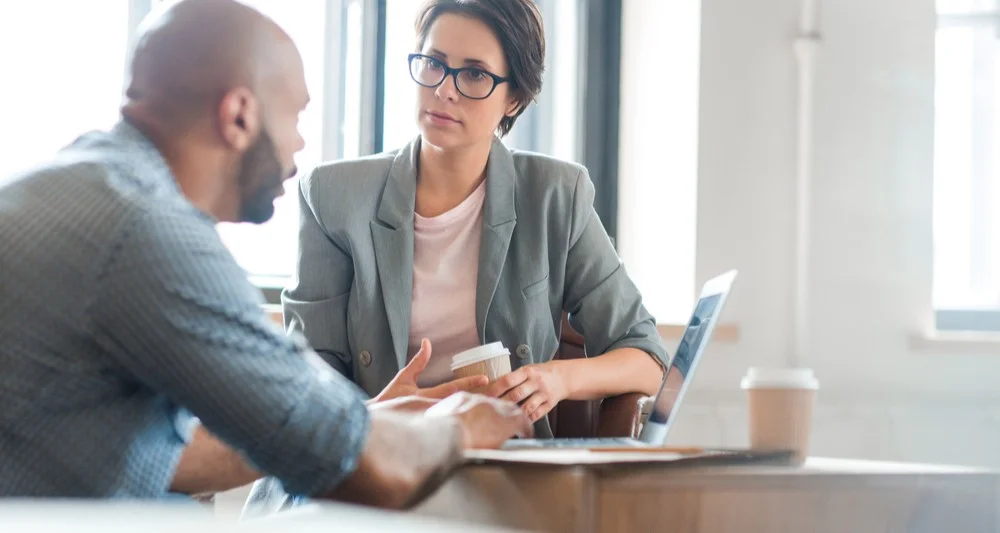